This study was conducted to understand the behaviour of ten rice genotypes for different water deficit stress levels. The spectroscopic hyperspectral reflectance data in the range of 350–2500 nm was recorded and relative water content (RWC) of plants was measured at different stress levels.
Techniques Adopted-
The optimal wavebands were identified through spectral indices, multivariate techniques and neural network technique, and prediction models were developed. The new water sensitive spectral indices were developed and existing water band spectral indices were also evaluated with respect to RWC. These indices based models were efficient in predicting RWC with R sq values ranging from 0.73 to 0.94.
Contour Plotting
The contour plotting using the ratio spectral indices (RSI) and normalized difference spectral indices (NDSI) was done in all possible combinations within 350–2500 nm and their correlations with RWC were quantified to identify the best index.
Multivariate Modelling and ANN
Spectral reflectance data was also used to develop partial least squares regression (PLSR) followed by multiple linear regression (MLR) and Artificial Neural Networks (ANN), support vector machine regression (SVR) and random forest (RF) models to calculate plant RWC. Among these multivariate models, PLSR-MLR was found to be the best model for prediction of RWC with R sq as 0.98 and 0.97 for calibration and validation respectively and Root mean square error of prediction (RMSEP) as 5.06.
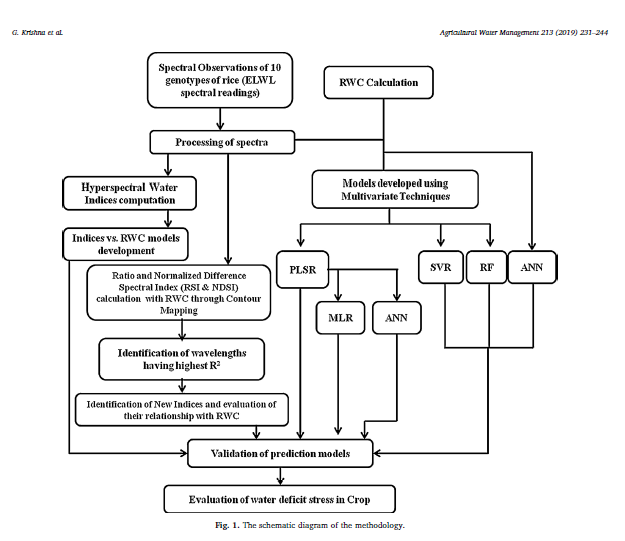
Results
The results indicate that PLSR is a robust technique for identification of water deficit stress in the crop. Although the PLSR is robust technique, if PLSR extracted optimum wavebands are fed into MLR, the results are found to be improved significantly.
The ANN model was developed with all spectral reflectance bands. The 43 developed model didn’t produce satisfactory results. Therefore, the model was developed 44 with PLSR selected optimum wavebands as independent x variables and PLSR-ANN model 45 was found better than the ANN model alone.
The study successfully conducts a comparative 46 analysis among various modelling approaches to quantify water deficit stress. The methodology developed would help to identify water deficit stress more accurately by predicting RWC in the crops.
Read Full Paper at the link given below-
Gopal Krishna
Doctoral Researcher,
Amity University, Noida,
India
Comments